In this Intellectual Production, my task was to analyze 12 hours and record what I paid attention to. This includes multi-tasking and how much attention I paid attention to the task. I logged Friday, February 23rd, 2024. This day happened to fall on our February break; therefore, I was not at work. The Scotties Tournament of Hearts (women’s curling) was on. My wife was curling in a local bonspiel in town, and we had some distractions from the ongoing teacher strikes and sanctions in Saskatchewan. We also have a very busy baby, who you will be able to see takes up much attention.
Over the 12 hours, I logged 42 different tasks across the time frame. I will also share my cell phone screen time, looking at the notifications I have received, the types of apps I used, the top apps I use, and the number of times I unlocked my phone. I will be the first to admit that my phone is an ultimate distraction and that I will spend more time on it than I would like to admit. Therefore, it would be essential to provide additional analysis on my cellphone usage over this time period.

Through my analysis of my attention, Citton (2017) highlights attention being a matter of selection. In attention economies, others are consistently vying for our attention. “Attention is a matter of selection, the positions of power in an attention economy are defined according to their ability to filter the flows that pass through us” (Citton, 2017, p. 178). This was interesting in my analysis. The advertisements and commercials that are presented within the Scotties Tournament of Hearts, as well as the flyers I received in the mail, passed through my filter. It begs the question, what are the consequences of what I opted to pay attention to.
Further analysis of my attention could be categorized into collective attention, joint attention, and individual attention (Citton, 2017).
Collective Attention
Collective is attention at the broadest scale. Within my day, this includes the engagement that I did with my Teacher Association group chat when we heard about province-wide sanctions that were going to be imposed on my first day back to work, although I may not have messaged back in my group chat, I gave attention to a significant number of people, as well as was mindful of the situation on a provincial scale. As well as my engagement with the Scotties Tournament of Hearts as well as viewing TikTok videos on my front page, which have been viewed thousands of times before I had even viewed them.
Joint Attention
Joint Attention refers to the attention of other specific people who are close to me. In my day, it was clear that this was a large majority of the day. I paid significant attention to my baby, my wife, and to a chat I had with a friend over Snapchat. Joint attention is critical for child development, thus reading to my child, and playing with my child is a major component of our days.
Individual Attention
Citton (2017) identifies individual attention as objects on which our bodies and spirits are nourished. For me, these were the tasks that I spent time on specifically for me. On this particular day, it included planning my law lessons for work, as well as washing my car, walking to go get the mail, and listening to some music.
Attentional Category
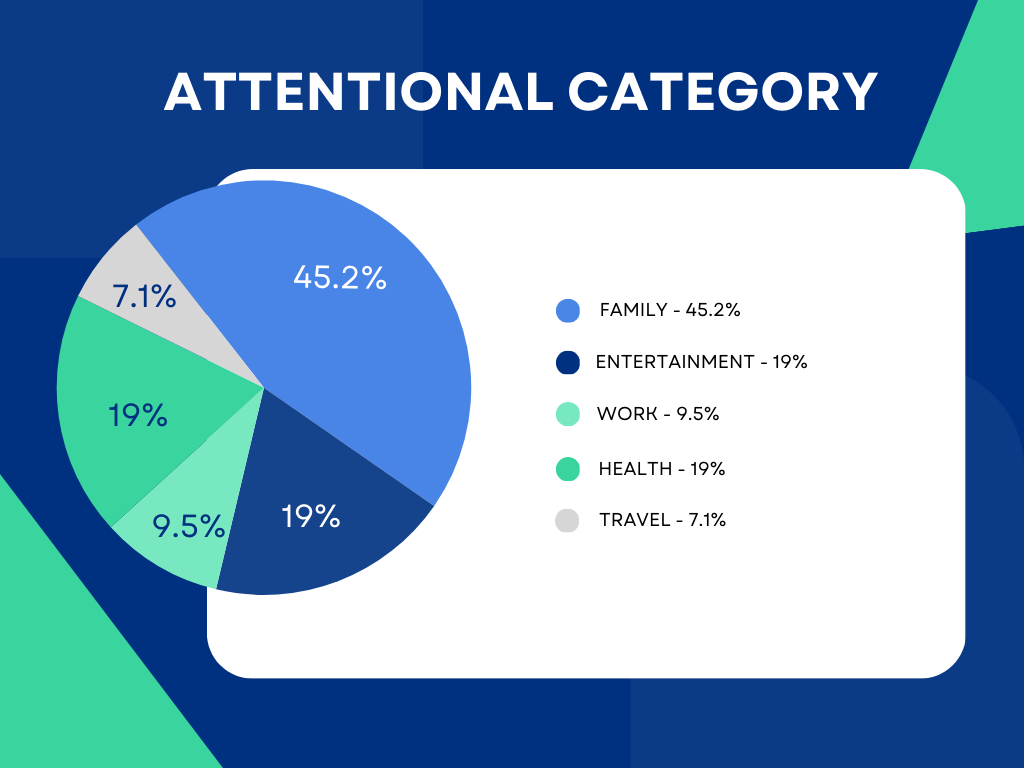

Within my analysis of my attention. I decided it would be important to see what I was valuing with my attention though the analysis of specific instances (e.g. “playing with baby” was coded as “family”). It was also important for me to see how long I was paying attention to the attentional objects. This analysis was interesting, as you can see that I have a total of 14 hours and 50 minutes. Often throughout the day, I would have been multitasking. The duration of my logged attention would have been logged twice. For example, watching curling on TV will playing on the floor with my baby is the reason why the entertainment sections of the graph are so different. While I had the TV on for an hour, passively paying attention, I also was doing other things, making breakfast, and playing with my child.
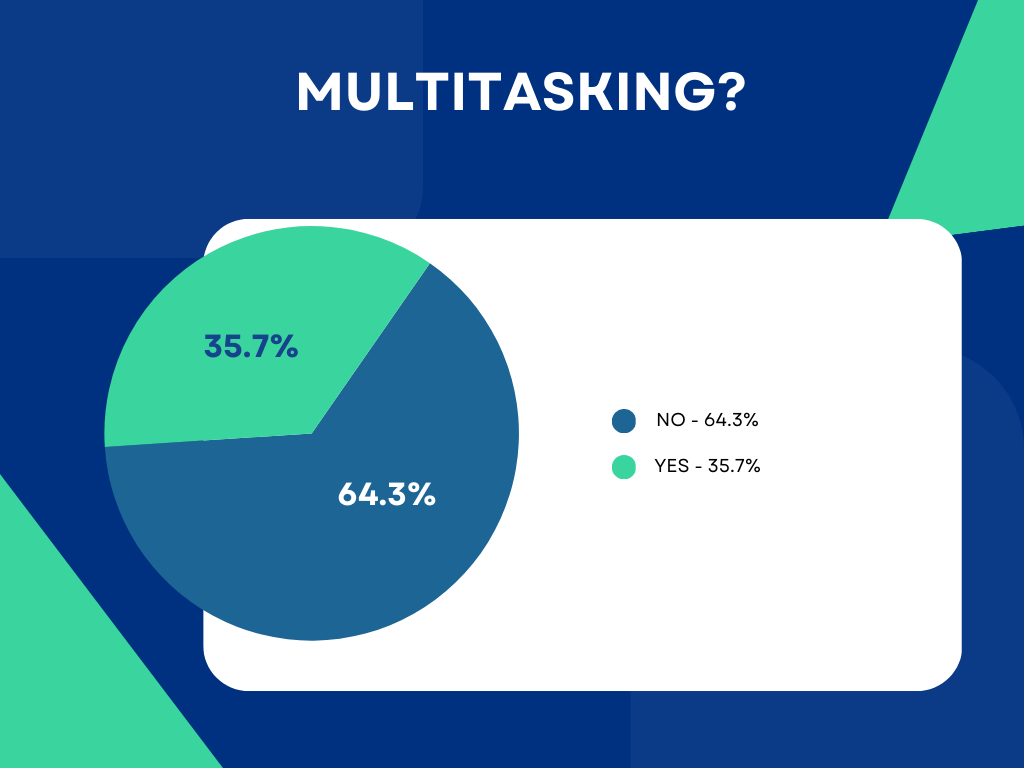
Attentional State
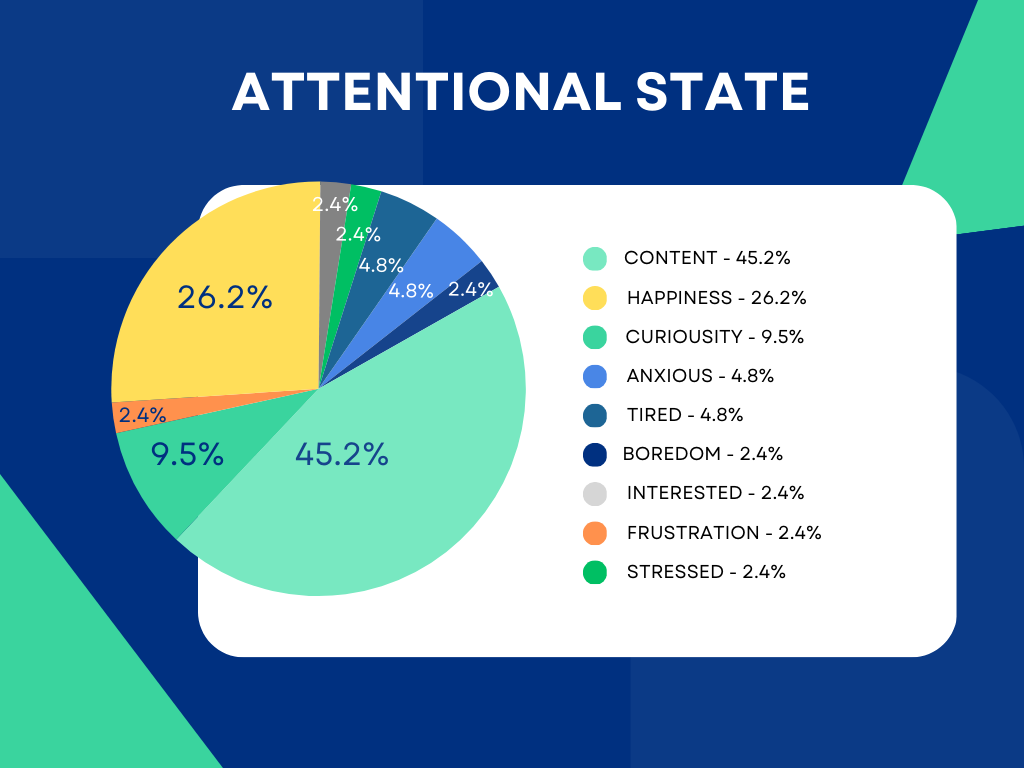
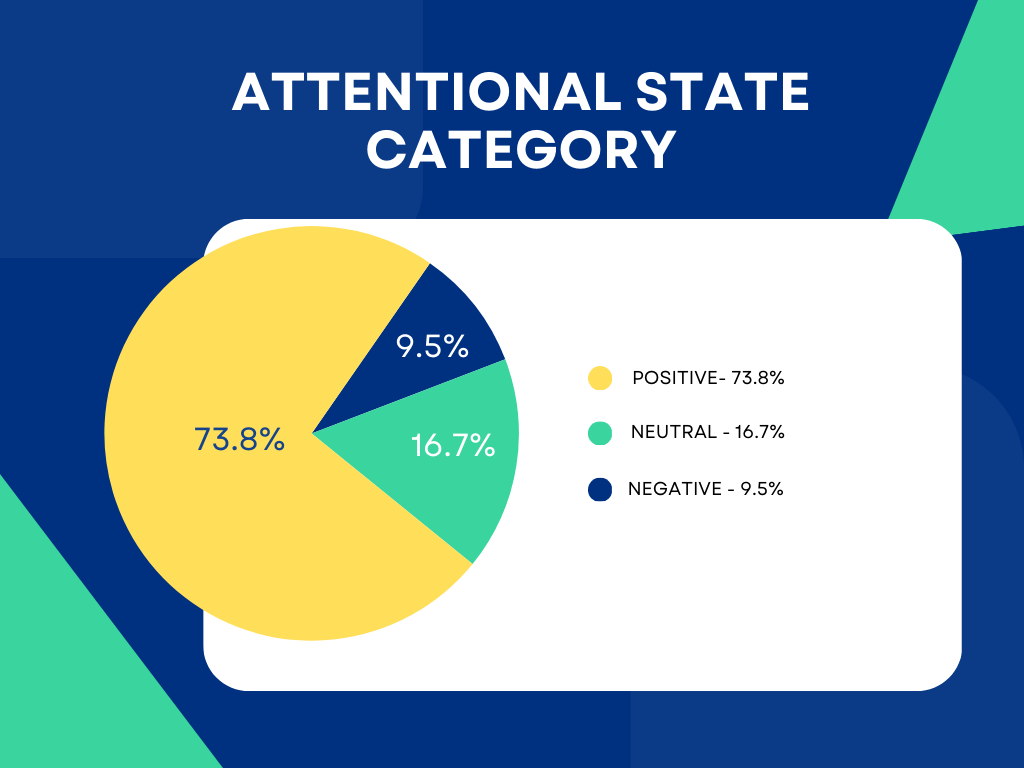
The analysis of my attentional state was first analyzed through how I was feeling while completing the task. These states were then further coded into whether the state was positive, neutral, or negative. The data highlights that a vast majority of my day was positive.
Voluntary or Automatic

Citton (2017) identifies that our automatic attention is the attention like hearing our name being spoken in a party. Within my analysis, I logged 9.5% of my attention as automatic in the 42 tasks tracked. This includes hearing notifications on my cellphone as well as the feeling of hunger. Voluntary attention is then the opposite, the times where I had to specifically focus my attention on specific tasks.
Cellphone Usage
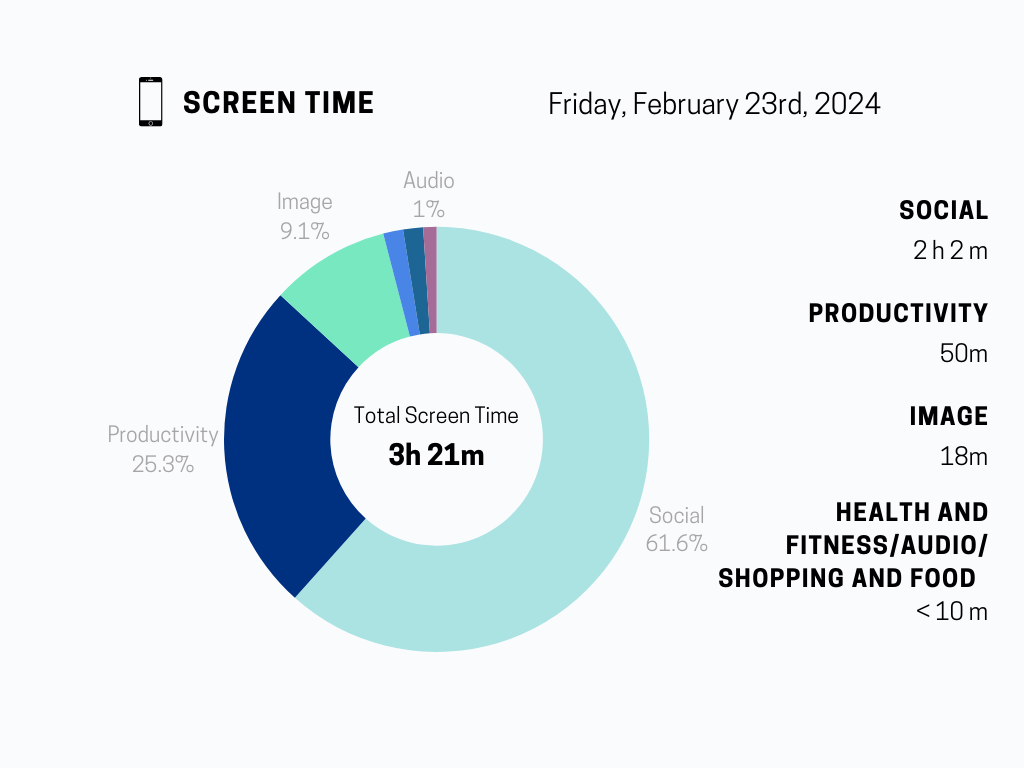
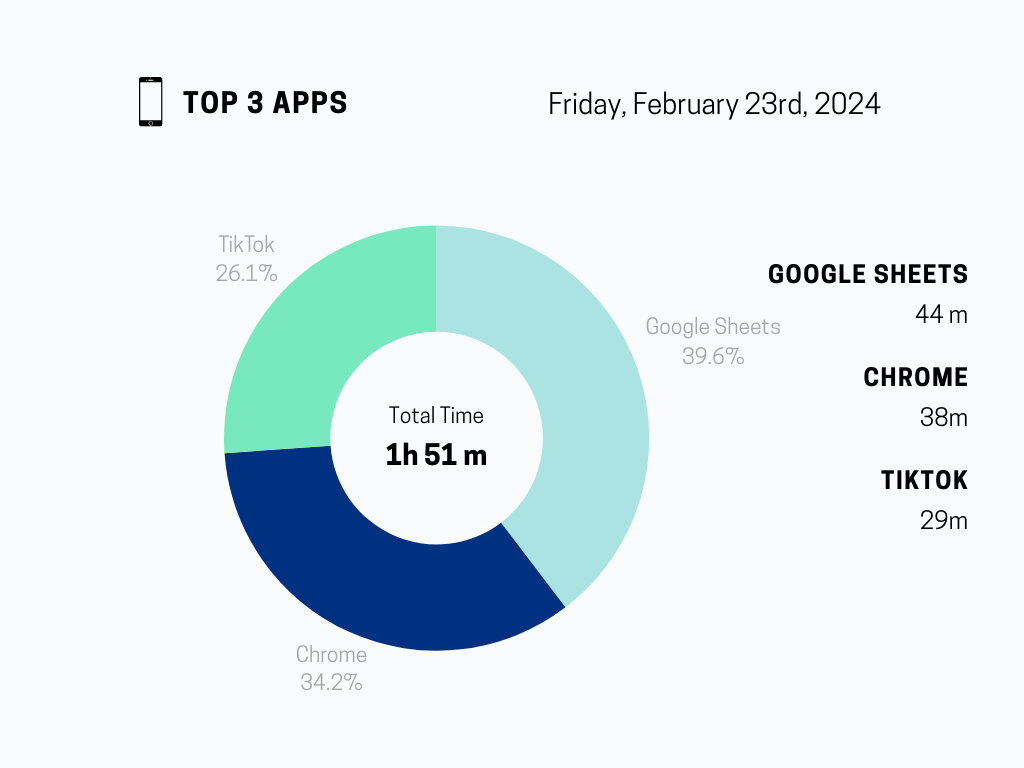
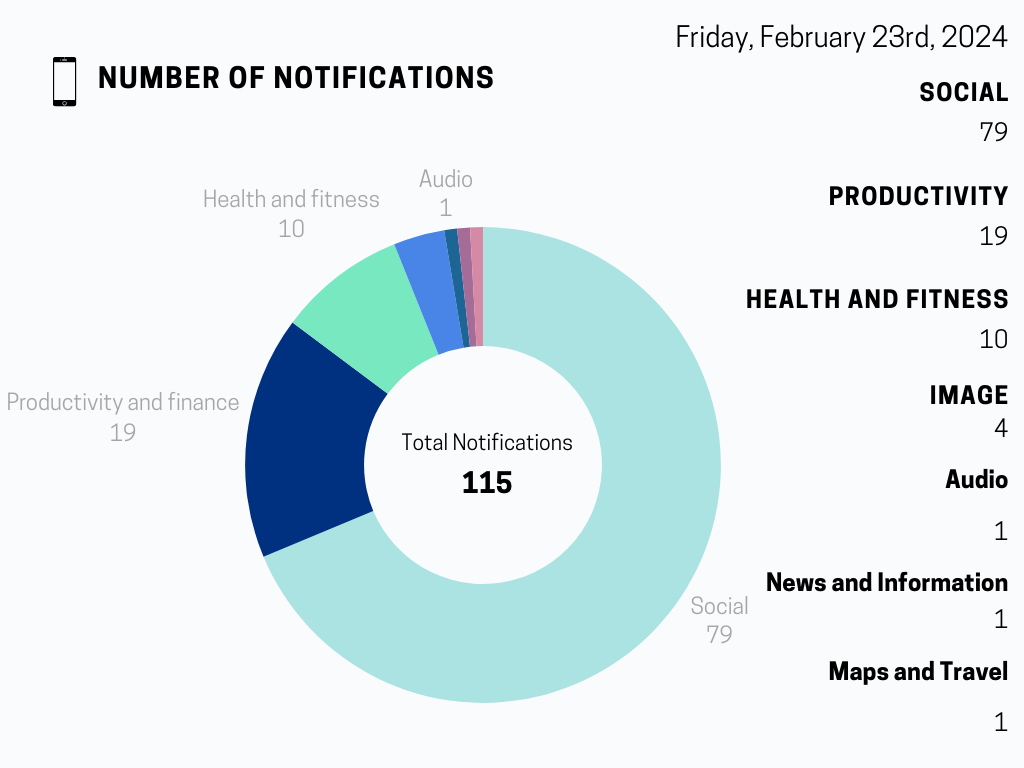

Throughout my day, you can see that I had 3 hours and 21 minutes of screen time. I know that I am often on my phone, but this was shocking to see, as it is not reflected well in my data that I have tracked. I think that this is partially due to my cellphone becoming a form of automatic attention, as compared to voluntary. Often when my phone does go off, I have to pause what I am doing and look at the notification. The notifications graph identifies the areas of which my attention is drawn. It is not surprising to see the correlation between social notifications and the amount of time spent on social apps. Often I will turn off notifications on my devices if I find them not helpful or unwanted.
Attention in Education
Within the topic of attention economies in education, we need to ask ourselves, how do we engage students? In looking at de Castell and Jenson (2004), the authors identify the cultural shift education has undertaken from teacher-centred to learner-centred. Thus, our shift from how we paid attention in school has also vastly changed our course. However, de Castell and Jenson discuss how video games and gaming, in general, can change the course of education through immersive experiences and their success in holding the user’s attention. Learning theories such as Seymour Papert’s constructionist approach, where students develop meaning through making, allow students to direct their attention in immersive opportunities with or without technology.
In addition, de Castell and Jenson (2004) identify that a reason why video games might be successful in holding student’s attention is that video games “help their players ‘learn’ quickly” (p. 396). Proper differentiation strategies that focus on individualized learning opportunities, such as the Modern Classroom Project, help teachers through blended instruction, self-paced structures, and mastery-based learning. This approach helps students maintain better attention because students can navigate at their own pace until they have mastered a topic.
References
de Castell, S., & Jenson, J. (2004). Paying attention to attention: New economies for learning. Links to an external site.Educational Theory, 54(4), 381–397.
Citton, Y. (2017). Introduction and conclusion: From attention economy to attention ecology. In Y. Citton, The ecology of attention. John Wiley & Sons.